The world of artificial intelligence (AI) is changing fast. Now, we search solutions that are affordable and secure. Open-source large language models (LLMs) are making this possible. They let individuals and businesses use AI safely and effectively. This article discusses how AI is free and secure.
These models can be used locally, keeping your data safe. They can be enhanced with features like Retrieval-Augmented Generation (RAG) and fine-tuning. These make the AI work better for you and keep your data secure.
Open-source AI is becoming popular. It’s cheaper and can be customized. By using LLMs locally, you control your data and keep it safe. RAG and fine-tuning let you make the AI work just for you, opening up new possibilities.
Key Takeaways
- Discover the benefits of free and open-source AI solutions for data privacy and security
- Understand the power of Retrieval-Augmented Generation (RAG) and how it can enhance the performance of your AI models
- Learn about the process of fine-tuning open-source LLMs to tailor them to your specific needs
- Explore affordable options for implementing private AI solutions in your organization
- Gain insights into the latest trends and developments in the world of open-source AI
Table of Contents
Unlock the Power of Free and Secure AI
The rise of open-source language models has opened up new possibilities in artificial intelligence. The web UI tools for local AI deployment using opensource LLMs, change how we use data to solve problems. They give us top-notch AI abilities without the high cost.
The Rise of Open-Source Language Models
The AI world has seen a big change with the open-source movement. Now, we have language models that are free and can be changed by anyone locally. Developers and researchers can use and improve these opensource LLMs. This lets them make new apps that fit their needs.
This has opened doors for all kinds of organizations. They can use advanced language processing without spending a lot of money.
Data Privacy and Security Concerns
As more people use AI, worries about data privacy and data security grow. Private AI solutions often send and store sensitive data online, making us question how it’s protected. Open-source language models offer a better choice.
They let organizations use AI on their own servers. This means they can keep a closer eye on their data. It ensures data privacy and data security.
Feature | Proprietary AI | Open-Source Language Models |
---|---|---|
Cost | High | Free or low-cost |
Data Control | Limited | High |
Customization | Restricted | Flexible |
Privacy and Security | Concerns | Enhanced |
The table shows the main differences between private AI and open-source language models. It points out the benefits of the latter in cost, control, customization, and data privacy and data security.
The growth of opensource LLMs offers a strong alternative. It lets organizations use advanced language models while focusing on data privacy and data security. By embracing this tech, businesses and developers can make innovative apps. These apps meet their needs without overcharging or risking security.
Introducing RAG: Retrieval-Augmented Generation
A new technique in artificial intelligence is making waves: Retrieval-Augmented Generation (RAG). It blends language models with the power to find relevant info from outside sources. This mix boosts data safety and helps the AI understand context better.
How RAG Works
RAG combines a language model and a retrieval model. The language model creates text that makes sense. The retrieval model looks through databases and websites for the right info. Together, they make the AI give answers that are both smart and right for the situation.
Benefits of RAG with LLM
RAG brings big benefits for keeping data safe. It uses info from outside sources to make answers more accurate and less biased. This lowers the chance of giving out wrong or harmful info.
This means the AI can handle new information and stay reliable. It’s great for situations where you need trustworthy info.
Fine-tuning Open-Source Language Models
In the world of artificial intelligence, fine-tuning open-source language models is a big deal. It lets you adjust these powerful models to fit your specific needs. This can bring a new level of accuracy and relevance to your projects.
Tailoring Models to Your Specific Needs
Fine-tuning open-source language models, like those based on the opensource LLM architecture, means you can make them fit your unique data and needs. You retrain the model with your own data. This helps it learn the specific details and patterns of your area.
The end result is a highly customized fine tuning solution. It does better than standard language models. Your outputs will be exactly what you need.
Benefit | Description |
---|---|
Improved Accuracy | By fine-tuning the model, you can significantly enhance its accuracy. This ensures it understands and generates content that is highly relevant to your needs. |
Customization | The fine-tuning process lets you customize the language model for your specific use case. It’s tailored to your unique data and needs. |
Enhanced Relevance | With fine-tuning, the model’s outputs will be more relevant and aligned with your target audience. This provides a more engaging and valuable experience. |
Whether you’re working on a customer service chatbot, a content generation tool, or any other application that uses natural language processing, fine-tuning open-source language models is a smart move. It’s a powerful and cost-effective way to boost your success.
“Fine-tuning open-source language models is a game-changer. It lets you unlock the full potential of AI for your unique needs.”
Setting Up a Local AI Environment
Starting with open-source language models for AI projects means setting up the right environment. This is key for developers, researchers, or enthusiasts. You need the right hardware and software for a smooth AI system setup.
Hardware and Software Requirements
You’ll need certain hardware and software for a strong AI environment:
- A powerful computer or server with a dedicated GPU (Graphics Processing Unit) for efficient model training and inference. The recommended hardware includes:
- CPU: Intel Core i7 or AMD Ryzen 7 (or higher)
- GPU: NVIDIA GeForce RTX 3080 or higher
- RAM: 32GB or more
- Storage: 1TB SSD for fast data access
- UI for local deployment of opensource LLM and RAG
By setting up your AI environment well, you can use open-source language models fully. This lets you deploy them securely and cost-effectively for your projects.
Common Pitfalls and How to Avoid Them
Installing and configuring open-source language models can be complex. Many organizations face issues with technical setup, like hardware and software requirements. To overcome this, following a detailed installation guide and using community resources for help is key.
Data privacy and security are big concerns when using AI. When handling sensitive data, making sure AI systems are secure and meet legal standards is crucial. Using Retrieval-Augmented Generation (RAG) can help by keeping data local and reducing the risk of data breaches.
The fine-tuning process can also be tough, needing a deep knowledge of the language model and the specific use case. Offering comprehensive training and support can help organizations get past these challenges. This way, they can customize their AI systems for their needs.
Increasing Accessibility and Adoption
Open-source AI is getting easier to use, which means more people and companies will start using it. Lowering the cost and complexity of these tools will make advanced AI more accessible to everyone. This will lead to more innovation and new ways to use AI, shaping its future.
The future of open-source AI is full of exciting possibilities. As new trends and developments come along, we’ll see more secure, customizable, and accessible AI tools. These tools will help people and organizations use AI to its fullest potential, changing the world.
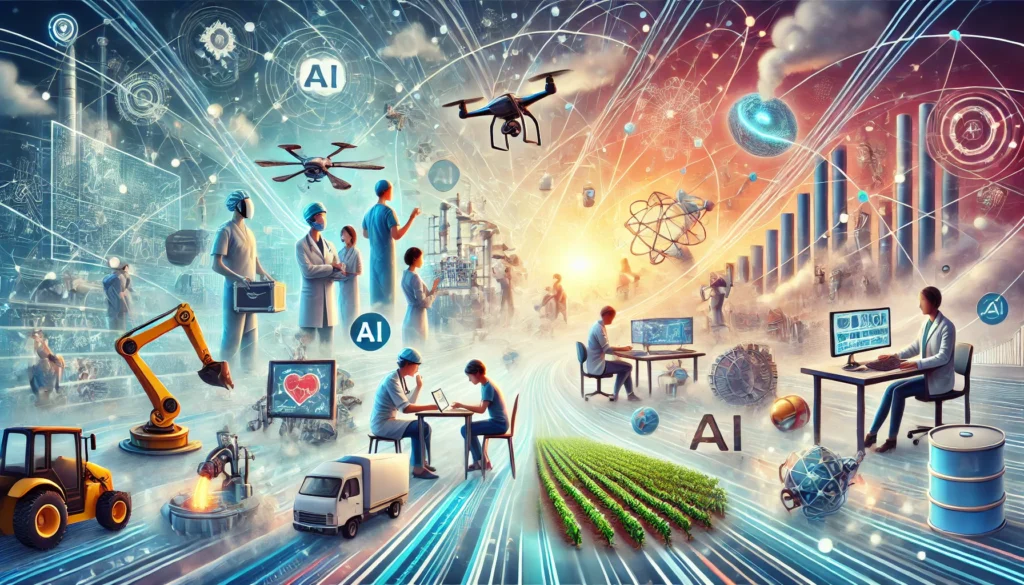
An abstract representation of an open-source AI ecosystem, where different tools and resources are interconnected and working together seamlessly to achieve a common goal.
Conclusion
In this article, we’ve looked at how free and secure open-source AI tools can change the game. We’ve seen how Retrieval-Augmented Generation (RAG) and fine-tuning language models can help. These tools offer affordable and customizable AI solutions that focus on keeping your data safe and private.
Setting up a private AI system has never been easier. This guide has shown you how to create a local AI setup and use the latest open-source language models. Whether you’re a business looking to improve or an individual curious about AI, the possibilities are vast.
The future of AI is exciting, with open-source and privacy-focused tools leading the way. By keeping up with these new solutions, you can be ahead of the curve. You’ll gain more efficiency, productivity, and security. Start this journey and see how free and secure AI can transform your world.
FAQ
What is the difference between open-source and proprietary AI solutions?
Open-source AI solutions, like large language models (LLMs), are powerful and don’t cost much. They don’t raise privacy concerns either when deployed and run locally. You can use them on your own, giving you more control and security over your data.
How does Retrieval-Augmented Generation (RAG) work?
RAG combines language models with the ability to find relevant info from outside sources. This makes AI responses more contextual and accurate. It also makes the AI safer by using your data more wisely.
What are the benefits of fine-tuning open-source language models?
Fine-tuning open-source language models lets you adjust them for your specific needs and data. This boosts the AI’s accuracy, relevance, and performance. It makes the AI work better for your unique needs.
What hardware and software are needed to set up a local AI environment?
You’ll need a strong GPU, enough RAM, and the right software tools for a local AI setup. This includes a Python runtime, package managers, and the open-source LLM framework you choose. The exact needs depend on how complex your project is.
What are some real-world applications of open-source LLM-based AI systems?
Open-source LLM-based AI systems are used in many areas. They help with natural language processing, content creation, answering questions, and more. They’re used in education, and customer service, among other fields.
What are the common challenges and limitations of deploying open-source AI locally?
Some challenges include limited hardware, optimizing models, and needing special skills for fine-tuning and deployment. There might be trade-offs in performance and the need for ongoing updates and maintenance.